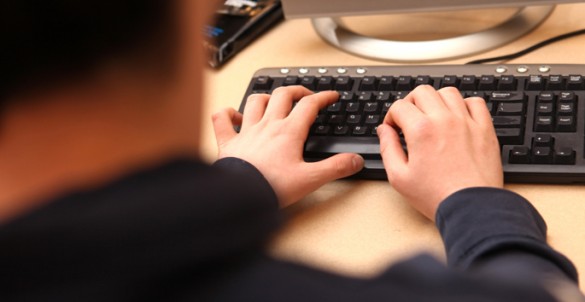
A Vanderbilt study shows that anonymization algorithms can provide privacy protection across multiple institutions as clinical data are released for research. (photo by Susan Urmy)
A new study, led by investigators at Vanderbilt University, confirms that the scientific pursuit of so-called big data from hospitals and clinics needn’t conflict with patient privacy.
As electronic medical records become standard, there is increasing interest in searching for patterns of health and illness in routine clinical data associated with genomic and other data derived from patient bio-samples.
The study, published in the Journal of the American Medical Informatics Association, is the first to demonstrate that a single patient anonymization computer algorithm can provide a standard level of privacy protection across multiple institutions as clinical data are released for research.
The study crucially shows that, at least where larger clinical datasets are concerned, patient anonymity requires little or no information generalization and suppression of the sort that would tend to spoil the utility of data for biomedical discovery.
“This is about recognizing that institutions are going to be increasingly pressured to share data from electronic medical records for research endeavors beyond their borders. This push is going to be led by efforts in ensuring reproducible research and combining data from institutions across the country to boost statistical evidence,” said Brad Malin, associate professor of biomedical informatics and associate professor computer science in the School of Engineering’s Department of Electrical Engineering and Computer Science. He also is director of the Health Information Privacy Laboratory.
For the study, Malin, computer scientist Raymond Heatherly, and colleagues posed a privacy adversary who has acquired patient diagnoses from a single unspecified clinic visit; to gain more complete knowledge, the adversary seeks to match these known data to a record in a de-identified research dataset known to include the patient.
From previous research using Vanderbilt data, Malin and his team understood that the more data that are associated with each de-identified record, and the more complex and diverse are the patient problems, the more likely information will stick out, requiring generalization or suppression to ensure anonymity.
The study used records from three institutions: VUMC, Northwestern Memorial Hospital in Chicago, and Marshfield Clinic, which has locations throughout Wisconsin.
“We didn’t know how our algorithm would play out at other institutions, where the patients seen and the usage patterns for standardized clinical terms, such as billing codes, might be different from those here at Vanderbilt,” Malin said.
To hide diagnosis code combinations that potentially could identify a patient, the team’s algorithm strips numbers off the back ends of codes to generalize them as needed, so that, with respect to single clinic visits, every record is surrounded by at least four doppelgängers. When this selective generalization fails to produce the required look-alikes, the algorithm suppresses codes altogether.
What the team wanted to know was how much generalization and suppression would be needed to achieve this standard level of anonymity and whether the protected data would be of any value for research.
The team processed relatively small datasets from each institution, representing patients in a multi-site genotype-disease association study, larger datasets representing patients in each institution’s bank of de-identified DNA samples, and large sets representing each institution’s electronic health record population.
Among the smallest three datasets (averaging 2,805 patients each), on average 12.8 percent of diagnosis codes required generalization; among the bio-bank-sized datasets, on average 4 percent of codes needed generalization; among the three largest datasets (averaging 533,324 patients each), no codes required suppression and on average only 0.4 percent required generalization.
While the study was limited to diagnosis codes, according to Malin, the results would be expected to hold for most types of clinical data.
“It was encouraging to discover this method is scalable; you could use the exact same strategy as an overlay on top of all hospital systems across the country, building data warehouses that are queryable with quantifiable privacy guarantees,” Malin said.
Federal policy says that institutions releasing clinical data for research can, upon consultation with a data privacy expert, use any patient de-identification method they think best, but they may be legally liable if the protection proves faulty. Malin says the policy has sown uncertainty and hesitancy, and that to support the flow of vital research data a national clearinghouse is needed to study and certify patient anonymization solutions.
Other Vanderbilt investigators involved in the study included Paul Harris, biomedical engineering research professor and professor of biomedical informatics, and Josh Denny, a physician and associate professor of biomedical informatics. They were joined by investigators from Northwestern University and Marshfield Clinic.
The grant was supported by the National Science Foundation and the National Institutes of Health (grants HG006385, HG006378, TR000135, HG006844, LM010685, GM105688, HG006389, HG006388, TR000150-05).