The MASI lab is collaborating on the development of deep learning algorithms for abdomen segmentation that leverage artificial intelligence to better understand and diagnose disease.
The MASI lab, affiliated with the Vanderbilt Institute for Surgery and Engineering, is working with a team from EnvoyAI, which aims to simplify access to new AI algorithms by providing direct access to physicians, including radiologists, for integration into existing workflows. The MASI team has, in effect, turned biomarkers it has generated from features of radiology studies into an application that can be utilized for prospective clinical research.
“The performance of our methods are better than traditional methods on accuracy and computational time,” said Yuankai Huo, a Vanderbilt PhD candidate in electrical engineering and member of the MASI lab.
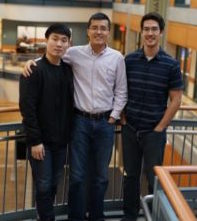
MASI (Medical-imaging Analysis and Statistical Interpretation), which is under the direction of Associate Professor Bennett Landman, is developing two algorithms with EnvoyAI. The first can produce 3D spleen segmentation from clinically acquired CT scans on both healthy people and symptomatic patients.
“This splenic biomarker is important because spleen size, morphology, and signal characteristics may be indicative of an underlying systemic disease processes,” said Dr. Steven Rothenberg, chief medical officer at EnvoyAI. “Currently, there is no standardized way to compare splenic size across patients and normative databases are very limited at the moment.”
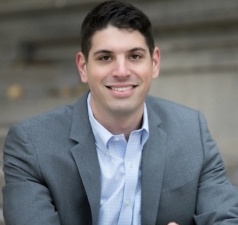
In other words, an enlarged spleen can be a sign of liver disease, infection and cancer. Variations in spleen size and shape on MRI images can result in large numbers of false positives and false negatives. The MASI lab’s approach to deep learning addresses limitations in methods that have been used.
Rothenberg demonstrated the software at the Radiological Society of North America (RSNA) 2017 annual meeting.
The second algorithm is a multi-organ segmentation pipeline, which can provide simultaneous liver, kidney, spleen and stomach segmentation on clinically acquired CT scans.
“The researchers at MASI have successfully turned their research biomarker into an application that can be used now for research purposes anywhere they grant permission,” Rothenberg said.
The underlying research is described in recent articles in SPIE Medical Imaging and IEEE Transactions on Biomedical Engineering.
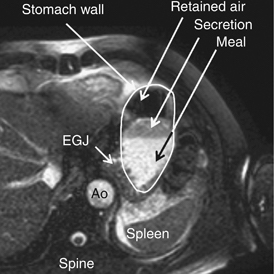
EnvoyAI, based in Cambridge, MA, launched its EnvoyAI Exchange in November. The cloud-based distribution platform allows access to algorithms without the need for underlying code or training data. The EnvoyAI platform “helps researchers and companies deploy their algorithms locally or in the cloud, while providing a distribution network for validation and commercialization,” Rothenberg said.
The company was formed in July 2017 after TeraRecon acquired McCoy Medical Technologies, a machine learning company, and spun out a new firm to provide simplified access to artificial intelligence algorithms that connect the work of individual end users, machine learning researchers, open source organizations and diagnostic imaging companies. It offers a developer platform and a vendor neutral API interface for integration.
The MASI lab is among several collaborators that include other AI companies as well as academic centers.