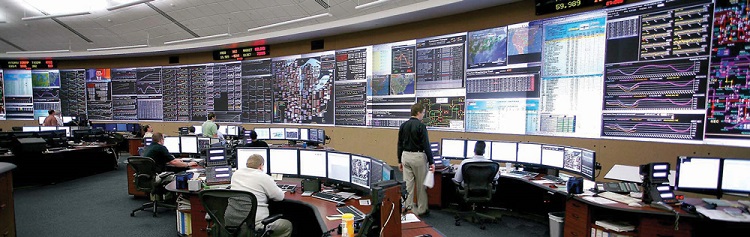
RISK, RELIABILITY AND RESILIANCE
Team will model time horizons from 15 minutes to 24 hours
The price for electricity on the wholesale market fluctuates every five minutes based on demand. Adding renewable energy to the mix greatly complicates matters. Historical methods that predict supply and demand are not accurate. Supply varies as the wind blows, or doesn’t, and as the sun shines, or doesn’t. Pricing electricity to maintain market equilibrium becomes a fast-moving target with ever more uncertainty.
Vanderbilt risk and reliability experts are part of a $3.25 million Department of Energy project that ultimately seeks to assign risk scores to energy assets to support quicker and more accurate market forecasts. The goal is to develop new machine learning algorithms that enable decision-making in near real time. The project is in concert with MISO, the Midcontinent Independent Supply Operator, a power consortium that operates the grid in all or parts of 15 states.
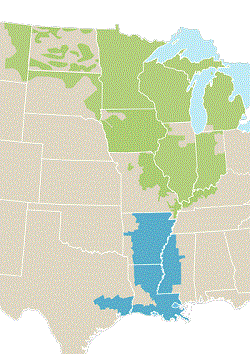
“The impact stands to be enormous,” said John R. Murray Sr. Professor of Engineering Sankaran Mahadevan. “This approach has the potential to transform how system operators plan and operate the U.S. grid, leveraging renewable energy sources, while minimizing the system risk.”
Mahadevan and Hiba Baroud, assistant professor of civil and environmental engineering and Littlejohn Dean’s Faculty Fellow, will lead Vanderbilt’s efforts. Their team will develop comprehensive risk metrics to support operational decisions that account for fluctuations and economic impact and handle statistical analysis of historical data to identify patterns and relationships among the many factors. They will use machine learning to speed up decision-making, based on optimum decision solutions for multiple scenarios generated by Georgia Institute of Technology, the project lead.
The goal of the project—Risk-Aware Market Clearing—is a blueprint for an end-to-end, data-driven approach that balances cost and minimizes system-level risk.
Market clearing is the process that keeps the supply level to the demand with no leftover of either.
Central to the project is a new statistical approach to forecast supply and demand. Historically the electricity market has relied on deterministic models, which predict outcomes with near certainty. Recent and fundamental advances in risk assessment, computational statistics, optimization and machine learning make it possible to use stochastic models that consider inherent randomness and uncertainty.
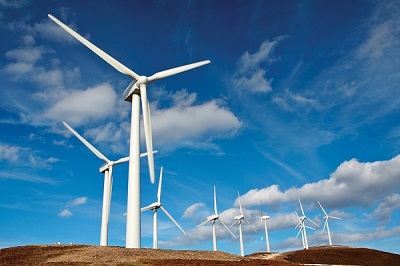
MISO, which acts much like an air traffic controller for the electric grid, has used traditional prediction models at three different time horizons: 24 hours, 15 minutes to three hours, and 10 minutes. Evolving factors that include randomness in renewable generation, load fluctuation with solar panels “behind” meters, and extreme weather increasingly affect all three predictions.
And that is with wind energy making up less than 20 percent of MISO’s energy portfolio. As the asset mix relies more on renewables and less on fossil fuels—MISO in February 2020 said it had projects totaling 57 gigawatts of solar power generation in the queue—forecasting risks escalate.
The team will use six years of historical data to build and train neural networks. The historical data covers:
- Renewable resource forecasts, including over 200 wind generators;
- Interchange and loop flows;
- Load forecasts for 38 Local Balancing Authorities;
- nGenerator non-compliance and status changes such as outages;
- Extreme weather events related to emergency conditions.
“We will leverage ideas developed in actuarial science to develop a composite risk metric that is similar to credit scores used in the financial industry, where we learn system-level risk based on many factors, individual asset risks and their correlations,” Baroud said.
The project is one of 10 funded by DOE’s Advanced Research Projects Agency as part of the Performance-based Energy Resource Feedback, Optimization, and Risk Management program.