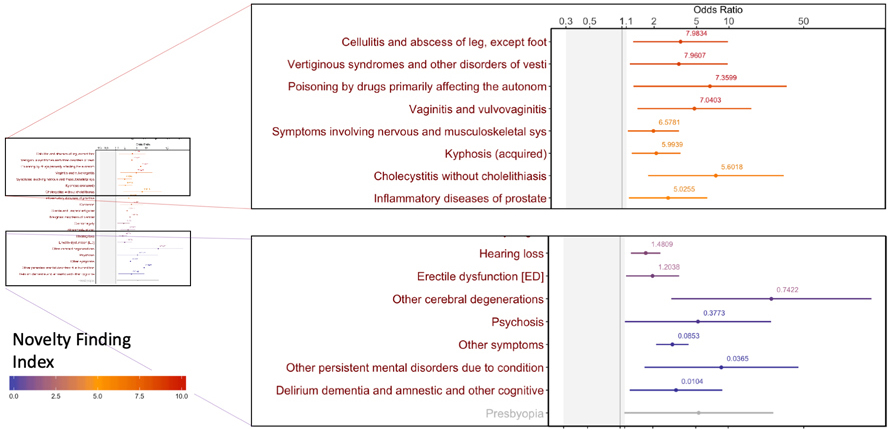
A new Vanderbilt-developed big data tool reveals novel associations between chronic diseases and lesser-known conditions that may help detect disease earlier and identify new research paths.
Using machine learning, anonymized electronic medical records, and peer-reviewed journal articles, a team of engineers, clinicians and informatic experts tested the tool for three conditions. In all three—Alzheimer’s Disease, Autism Spectrum Disorder and Optic Neuritis—the tool found lesser-known conditions that may support earlier monitoring or medical intervention. The novel associations also provide potential new insights into disease progression.
“We are excited about the opportunities to discover new risk factors and associations of diseases in the clinical record,” said Bennett Landman, professor of electrical engineering, computer engineering and computer science.
“Overall, our goal is to advance engineering and clinical science to improve the understanding and care of patients,” said Landman, who led the group.
For the project, researchers used de-identified EMRs of patient groups with each of the three conditions and appropriate control groups with comparable demographics but without the disease diagnosis. They mined real-time journal article abstracts from PubMed, a search engine maintained by the U.S. Library of Medicine, because well-known associations likely will have
more papers published about them than novel ones, said Shikha Chaganti, Ph.D’19, first author and a former student in Landman’s Medical-image Analysis and Statistical Interpretation Lab.
The algorithms searched for and tallied mentions of associations to each condition from article headlines, abstracts and keywords, said Chaganti, who is now at Siemens Healthineers as a senior deep learning research scientist.
The resulting tool, Phenome-Disease Association Study, or PheDAS, performs association studies and identifies disease comorbidities across time. It also solves a thorny issue for these types of studies: how to prioritize apparent correlations for clinical relevance. PheDAS correctly identified well-known associations with each of the three target conditions. But some associations will be so random they are likely to be unrelated or have extremely limited relevance.
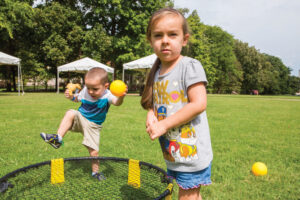
A “Novel Finding Index” guides researchers to significant associations that may be clinically relevant but have not been well-studied in medical literature. The index gives well-known disease associations a low ranking.
In the case of Alzheimer’s, for example, well-known associations identified included psychosis, cerebral degenerations and gait abnormalities and were given a low novelty score. Infections and inflammatory processes across several organ systems were among the novel associations identified in the five years prior to diagnosis and received higher scores.
“Our results demonstrate wide utility for identifying new associations in EMR data that have the highest priority among the complex web of correlations and causalities,” the team concluded.
The team made the tool free and available to the public online. The new tool kit, with its machine learning algorithms, creates easier, user-friendly access to a daunting amount of data.