Warning: This video has graphic images of surgical procedures.
An interdisciplinary fellowship with the Data Science Institute has resulted in a promising machine-learning technology that can effectively track complex surgical activity, thus having the potential to improve patient outcomes, safety and documentation.
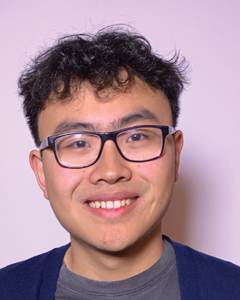
TingYan “Nicholas” Deng, a third-year student majoring in computer science, mathematics and economics, used algorithms similar to those that control autonomous vehicles to develop technology that analyzes surgical video captured by a camera worn around a surgeon’s neck.
The project was developed with Benoit Dawant, professor of electrical engineering and computer science and director of the Vanderbilt Institute for Surgery and Engineering, and Alexander Langerman, associate professor of otolaryngology – head and neck surgery, and a 2020 VISE Physician-in-Residence.
“Video is the ultimate objective record of what happens in the operating room,” Langerman said. “If a patient needs a second procedure, the surgeon can see exactly what happened during the first surgery. Thinking even bigger, surgical video can identify ways to improve surgeon performance and the elements that affect patient outcomes. We just need to make sure we’re capturing the right things.”
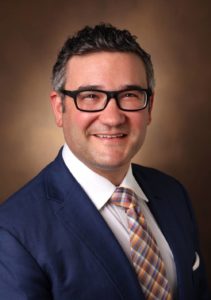
Deng’s work took on the next step of improving surgical video: ensuring that the camera is always aimed at the right spot.
The article “Automated detection of surgical wounds in videos of open neck procedures using a mask R-CNN” was published in the Conference Proceedings of the Society of Photo-optical Instrumentation Engineers on Feb. 15. This work is the first known demonstration of open surgical wound detection using first-person video footage.
Deng trained an algorithm called “mask R-CNN” on surgical videos to segment and track a surgical wound while being immune to distractions from the many hands, instruments and materials constantly altering light conditions and obscuring the field of view. This constant activity made applying mask R-CNN a difficult and highly technical challenge. After working with more than a thousand images, mask R-CNN can quantify the relative distance and movement between the wound, the surgeon’s hand and surgical instruments.
“For using a relatively small number of videos, the algorithm performs really well,” Langerman said. “I am confident that we are on the way to creating a highly reliable technique for detecting key elements of the open surgical field.”
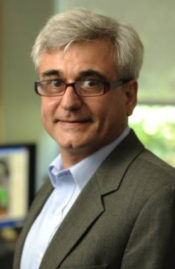
“This collaboration had very interesting components. TingYan brought his creative and determined attitude with him in developing mask R-CNN,” Dawant said. “We are optimistic about where this work is headed.”
Beginning this work in January 2020, Deng did not have much experience with machine- and deep-learning techniques. He feels he got a much more concrete sense of computer science during workshops hosted by the Data Science Institute and was able to apply those lessons to learn other algorithmic formations. From this experience, Deng has become interested in pursuing a data science graduate degree.
“The coolest part of this project is its interdisciplinary nature,” Deng said. “It is not easy to work with medical images because most are not openly available. I was very excited to participate in such a unique project, bringing innovative driving algorithms to surgery.”
Deng’s research was supported by VISE. He is working on two academic papers: one comparing mask R-CNN results and methodologies to existing approaches and another on a second object detection algorithm to track surgical instruments. Deng will continue his work through a VISE fellowship this summer.
By Marissa Shapiro
Contact: Brenda Ellis, 615 343-6314
brenda.ellis@vanderbilt.edu