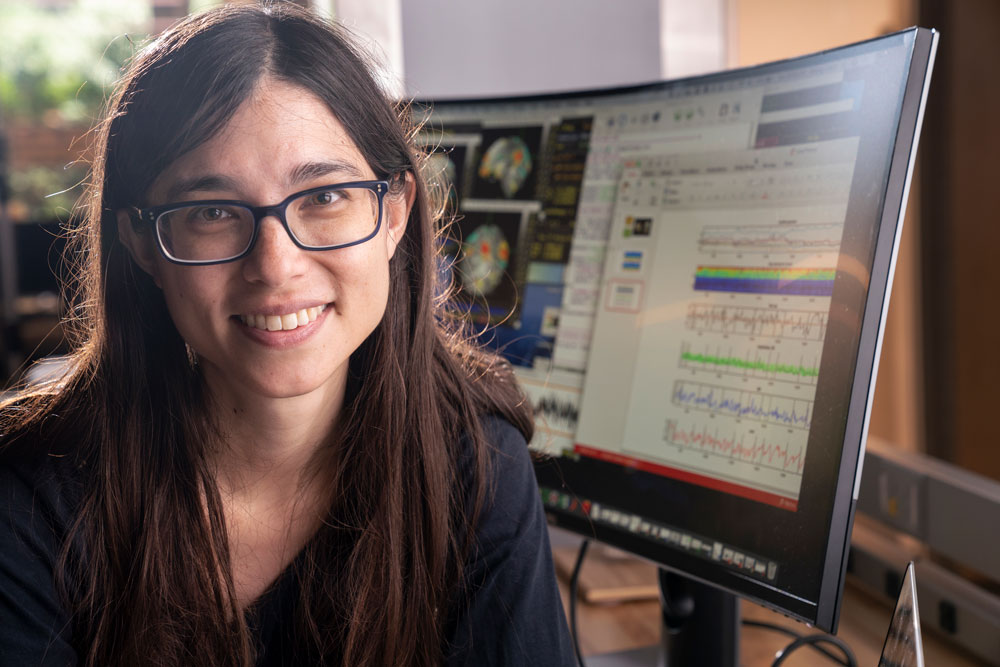
Advances in neuroimaging over the past 25 years have ushered in nothing short of a revolution in technology for understanding the human brain. These new technologies have opened broad vistas for scientists, from being able to pinpoint regions of the brain responsible for various functions and behaviors to targeting new treatments for illnesses ranging from depression to Parkinson’s disease.
At a deeper level, however, scientists lack a full picture of the fundamental network structures and mechanisms that govern not just our brains, but their interactions with many of the processes that take place throughout our bodies.
Writing in a Harvard Health blog post, Dr. Robert H. Shmerling underscores how difficult it is to discern the mechanics underlying certain traits and inclinations: “If you performed a CT scan, MRI scan, or even an autopsy on the brain of a mathematician and compared it to the brain of an artist, it’s unlikely you’d find much difference. And if you did the same for 1,000 mathematicians and artists, it’s unlikely that any clear pattern of difference in brain structure would emerge.”
Catie Chang, a multidisciplinary Vanderbilt scientist who holds faculty appointments in electrical and computer engineering, computer science and biomedical engineering, is part of a growing field of scientists who suspect there are deeper patterns in the brain to be discovered.
To find them, however, will require new methods of computational analysis and machine learning.
Like others using powerful computers to reveal hidden patterns in complex data like weather systems, financial markets or human vital signs, Chang is captivated by what the typically discarded “noise” in fMRI datasets may be able to tell us about the brain.
Functional MRI (fMRI) technology has been used primarily to shed light on how the brain responds to particular stimuli, whether subjects are being asked to solve a math problem or being shown images designed to provoke an emotional response. Similarly, EEG technology measures electrical activity in the brain, whether it’s while we’re sleeping or engaged in some cognitively demanding activity.
Both technologies capture vast amounts of background data that tends to be disregarded in many research studies. But to Chang and others, this information may contain tantalizing clues that could revolutionize our understanding of the brain.
“Neuroimaging technology has come very far,” Chang says. “But we’re still left with a ton of data that holds much untapped potential.”
For Chang, the possibilities are enticing. She says one avenue for the field is to use data-driven approaches to make new discoveries. Another is to employ machine learning to uncover biomarkers that could be used to make more accurate health predictions.
In addition to searching for direct clinical outcomes, she says, these new advances in computational analysis will ultimately give scientists a deeper, more precise understanding of how the brain works.
“The field has been asking questions like, how does the ongoing, background activity of the brain reflect and shape how people perceive things, or how people remember things,” Chang explains. “As the field advances, there’s more and more that we’re finding in all of this data.”
DUAL INTERESTS LEAD TO SINGULAR FOCUS
Chang’s own path to this intersection of computation and neuroscience began with an early fascination of biology. Growing up, she hadn’t been exposed to computer programming or engineering, but then as an undergraduate at MIT, she became captivated by her computer science classes and ended up majoring in the subject. Yet she never let go of her affinity for the biological sciences and was particularly interested in the brain.
As a student, she worked in what was then MIT’s standalone Artificial Intelligence Lab (it has since been absorbed into a wider lab, CSAIL) on an area known as biologically inspired computing. “It was trying to take biological principles and figure out how they can improve computing,” Chang says. “For instance, we looked at things like how cells—which are these distributed agents with no central command—grow in the body, and then explored how to replicate that in computers.”
By the time she began graduate school at Stanford, Chang once again found herself drawn to neuroscience, but always with a computational element involved. She performed research in a lab that was using computational methods to understand data from noninvasive neuroimaging technologies like fMRI and EEG. “That’s the research area in which I ended up continuing my Ph.D.”
Following graduate school, Chang worked as a postdoctoral and research fellow at the National Institutes of Health before joining Vanderbilt’s faculty in 2018. Today, Chang’s Neuroimaging and Brain Dynamics (NEURDY) Lab is an active member of the Vanderbilt Institute for Surgery and Engineering (VISE).
And in 2019, Chang and Dr. Dario Englot, Vanderbilt University Medical Center surgical director of epilepsy and associate professor of neurological surgery, electrical engineering, radiology and radiological sciences and biomedical engineering, received a $3 million NIH grant to study disturbances in brain networks in patients with epilepsy. That work continues today, as the team uses a combination of EEG and fMRI data to explore whether network disturbances caused by epilepsy contribute to cognitive deficits.
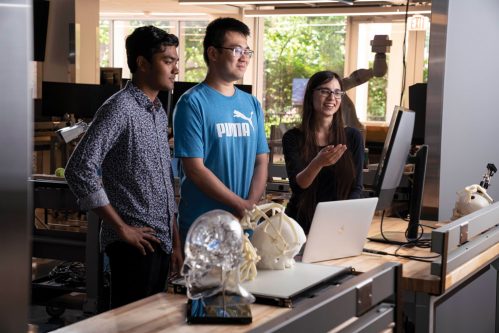
Although much work in the field seeks to quantify specific brain networks and explain how they operate, more recent efforts have sought to extend that understanding by looking at how these networks fluctuate over time or in different states of consciousness. This concept forms a key piece of the team’s work on epilepsy, and Chang is expanding this work to other neuroscience and clinical research areas as well.
“In this work, we can often take inspiration from spatiotemporal data and models from other fields and build upon these approaches to search for new insights in brain activity datasets,” Chang says. She mentions a recent collaboration with researchers at UCLA and Emory University that found a series of patterns in brain study data that are thought to constitute a set of building blocks that may link together what other researchers are observing in their studies—toward a kind of unifying model for the fundamental activity patterns in our brain.
“This work points to a common set of patterns that seem to account for a lot of the observed dynamics in the brain,” Chang says.
By its nature, Chang’s work is inherently multidisciplinary. Members of her lab come from areas ranging from biomedical and electrical engineering to physics and computer science. Similarly, research collaborators from across Vanderbilt include researchers from Peabody College, psychology, the Institute for Imaging Science and biostatistics.
“The work that Catie’s lab is doing—not just at VUMC, but across the field—could help revolutionize our collective understanding of how the brain works in an interconnected way with many different systems throughout the body,” says Bennett Landman, chair of the Department of Electrical and Computer Engineering, whose own expertise focuses on using computer-based statistical analysis to improve medical imaging technology. ”Her work is also emblematic of the kind of robust, interdisciplinary research collaboration that Vanderbilt fosters. It’s this kind of environment where true innovation can thrive.”