A front-end lens, or meta-imager (see below), created at Vanderbilt University can potentially replace traditional imaging optics in machine-vision applications, producing images at higher speed and using less power.
The nanostructuring of lens material into a meta-imager filter reduces the typically thick optical lens and enables front-end processing that encodes information more efficiently. The imagers are designed to work in concert with a digital backend to off-load computationally expensive operations into high-speed and low-power optics. The images that are produced have potentially wide applications in security systems, medical applications, and government and defense industries.
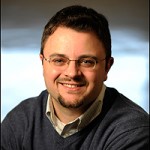
Mechanical engineering professor Jason Valentine, deputy director of the Vanderbilt Institute of Nanoscale Science and Engineering, and colleagues’ proof-of-concept meta-imager is described in a paper published Jan. 4 in Nature Nanotechnology.
Other authors include Yuankai Huo, assistant professor of computer science; Xiamen Zhang, a postdoctoral scholar in mechanical engineering; Hanyu Zheng, Ph.D.’23, now a postdoctoral associate at MIT; and Quan Liu, a Ph.D. student in computer science; and Ivan I. Kravchenko, senior R&D staff member at the Center for Nanophase Materials Sciences, Oak Ridge National Laboratory.
This architecture of a meta-imager can be highly parallel and bridge the gap between the natural world and digital systems, the authors note. “Thanks to its compactness, high speed and low power consumption, our approach could find a wide range of applications in artificial intelligence, information security, and machine vision applications,” Valentine said.
The team’s meta-optic design began by optimizing an optic comprising two metasurface lenses which serve to encode the information for a particular object classification task. Two versions were fabricated based on networks trained on a database of handwritten numbers and a database of clothing images commonly used for testing various machine learning systems. The meta-imager achieved 98.6% accuracy in handwritten numbers and 88.8% accuracy in clothing images.
The research is supported by the Defense Advanced Research Projects Agency (DARPA), Naval Air Systems Command (NAVAIR), ONR and the National Institutes of Health (NIH).
contact: brenda.ellis@vanderbilt.edu