Kolouri wins $1M DARPA grant to investigate AI cooperative lifelong learning
A Vanderbilt engineering professor is leading part of an international initiative to create advanced artificial intelligence programs that will enable machines to learn progressively over a lifetime and share those experiences with each other. Researchers hope the technology will allow machines to reuse information, adapt quickly to new conditions and collaborate by sharing information.
Soheil Kolouri, assistant professor of computer science, in partnership with Hamed Pirsiavash, associate professor of computer science at the University of California, Davis, will lead a research team focusing on continual machine learning mechanisms.
The prototype project, “Information Distillation for Embodied and Articulate Lifelong Learners,” or IDEALL, has received a $1M award from the Defense Advanced Research Projects Agency as part of the agency’s Shared-Experience Lifelong Learning (ShELL) initiative. DARPA wants to develop AI agents that share their experiences with each other and is seeking innovative basic or applied research concepts in lifelong learning.
In addition to leading IDEALL, Kolouri’s team has partnered with Andrea Soltoggio, associate professor of computer science, University of Loughborough, UK, to develop a theoretical framework that allows AI agents to measure tasks’ similarities and continually learn by analogies. Cong Liu, associate professor of computer science, University of Texas, Dallas, is a member of Loughborough team.
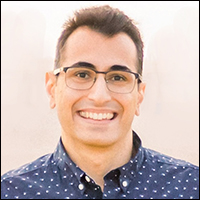
The Vanderbilt-UC, Davis team will concentrate on the algorithmic theory and statistical foundation of the learning mechanisms. The UK team will focus on novel bio-inspired neural networks that learn shareable knowledge exploiting neuromodulation and synaptic consolidation mechanisms, and the Texas researchers will focus on the hardware integration and deployment for potential transition to industrial and real-world applications.
The real-world uses of this new technology could include cooperating self-learning autonomous vehicles such as self-driving cars, robotic rescue and exploration systems, distributed monitoring systems to detect emergencies, or cyber security systems of agents that monitor large networks.
Lifelong Learning is a relatively new area of machine learning research in which agents continually learn as they encounter varying conditions and tasks while deployed in the field, acquiring experience and knowledge and improving performance on both novel and previous tasks. This differs from the train-then-deploy process for typical ML systems.
LL is an emerging area of machine learning that differs from the traditional train and then deploy process. In LL, an AI agent must continually learn from the input data stream while preserving and improving its previously acquired knowledge.
“Lifelong learning from the never-ending stream of everchanging data is the key to scaling up AI systems,” said Kolouri. “One of the major roadblocks in achieving LL is the so-called plasticity-stability trade-off, where plasticity refers to the ability to learn from new data, and stability refers to retaining the previously learned knowledge.” A team of undergraduate and graduate students at the Machine Intelligence and Neural Technologies (MINT) Lab directed by Kolouri, in collaboration with computer science associate professor Vladimir Braverman’s group at Johns Hopkins University, is currently studying this phenomenon.
“Today we know the importance of social interactions in the evolution of human intelligence. Artificial General Intelligence (AGI) could not be realized with a single AI agent. Similar to the cognitive revolution in sapiens, a transition is needed from our current single-agent LL to articulate LL machines that can encode information about their surroundings into a compact compositional language and use it for machine-to-machine communication and, maybe more importantly, for thinking, which is a form of self-communication!” said Kolouri. “The ShELL program aims to develop such communicative LL agents that continually learn from their collective experiences.”
“We are very excited to be part of this fast-paced, innovative program and look forward to transitioning our developed tools into medical applications,” Kolouri said.
The funding is part of DARPA’s Artificial Intelligence Exploration (AIE) program, a streamlined research and development opportunity aimed at encouraging quick innovations in rapidly acceleration technology.
Contact: Brenda Ellis, 615 343-6314
brenda.ellis@vanderbilt.edu