To shoot a basketball with precision requires countless hours of practice. Usually, this happens under the watchful eye of a coach, who can provide guidance on the right mechanics of each shot. Now, though, thanks to new research from Vanderbilt University, players may soon be able to use artificial intelligence technology to work on those same principles on their own.
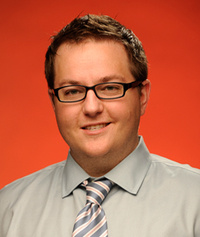
Jules White, associate dean for strategic learning programs and associate professor of computer science and computer engineering, and Carlos Olea, a Ph.D. student in the Department of Computer Science, analyzed machine learning to perfect basketball shot types in their award-winning conference paper, “Analysis of Deep Learning Action Recognition for Basketball Shot Type Identification.”
“There’s a role for artificial intelligence to play in sports,” White said, “because there are numerous opportunities to take data and provide that data to individuals to help them improve their game.”
White and Olea worked with NOAH Basketball, an organization that uses cameras and cutting-edge software technology to calculate shooting statistics for NBA and NCAA players during practice. Through facial recognition and computer vision, NOAH software can identify who is shooting and provide athletes with unique interactive shot charts and data, including arc, depth, shooting percentages and consistency. In total, NOAH provided more than 50,000 hours of video footage, which White and Olea then used to classify different shot types.
Basketball, however, doesn’t always offer clean lines of sight for actions taken while playing, so creating the framework to understand what differentiated one shot from another presented a challenge for the researchers.
“The next level NOAH wanted to see is this idea that you get more than just where you were and whether or not you made it in the directory of the shots,” White said. “But also, under what conditions—like whether you just received a pass from the left or right.”
“We had to create a dichotomy of shot types we were looking at due to having so many variations within the sport,” Olea added. “No one shot is the same.”
Employing AI software called a temporal relational network, the researchers were able to optimize shot type recognition, achieving an accuracy of 96.8 percent on 1,500 novel shots. In the future, White and Olea hope that this study will assist in the enhancement of solo practices. This research has the potential to be executed through the use of their proposed five shot type dichotomy as a functional tool within an app or website with the ability to show athletes the “correct” or a “better way” to take it to the hoop.
“I can think back to times where I’d be in my backyard, and I’d record myself doing something particular, making a throw or throwing a pitch or something like that and try to look back at that video feedback to try and see what I was doing wrong,” Olea said. “So having technology available to provide feedback, like a surrogate coach, is compelling not only for professionals but also for amateurs—whoever wants to try and improve at the sport.”
By: Celeste Malone